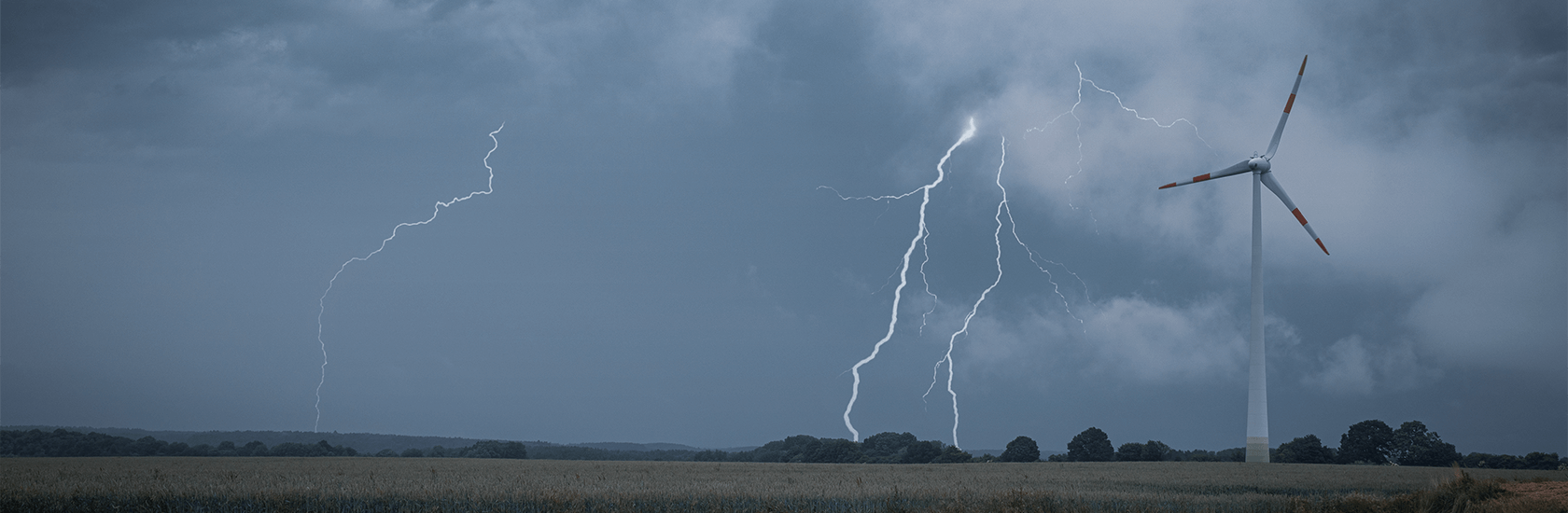
Catastrophic risk modelling insights
Inconsistent and poor quality data can hamper the accuracy of insurance risk models. Unstructured reports, incomplete information and duplicate records mean that data sources can not be efficiently incorporated into the analysis.
Clir leverages artificial intelligence, and anonymous claims and operations data, for improved risk modelling. Machine learning labels and enriches risk data to improve the quality, enabling a deeper understanding of project-specific risks and robust mitigation strategies.
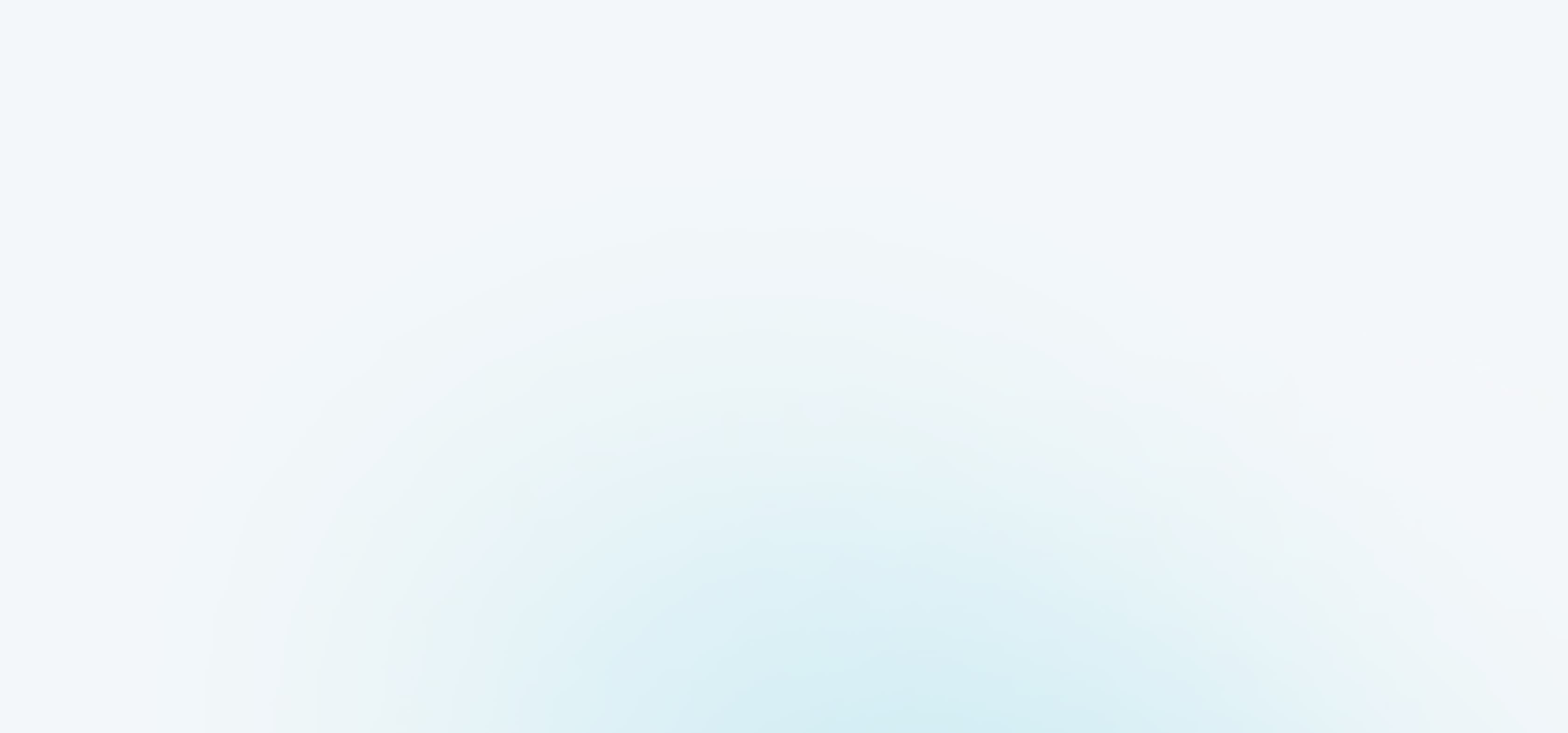
Risk modelling data includes
Insurance claims and policies to examine the claims history of assets with similar characteristics.
SCADA and event data to quantify business interruption loss expectations, and model the impact of downtime on property-related events.
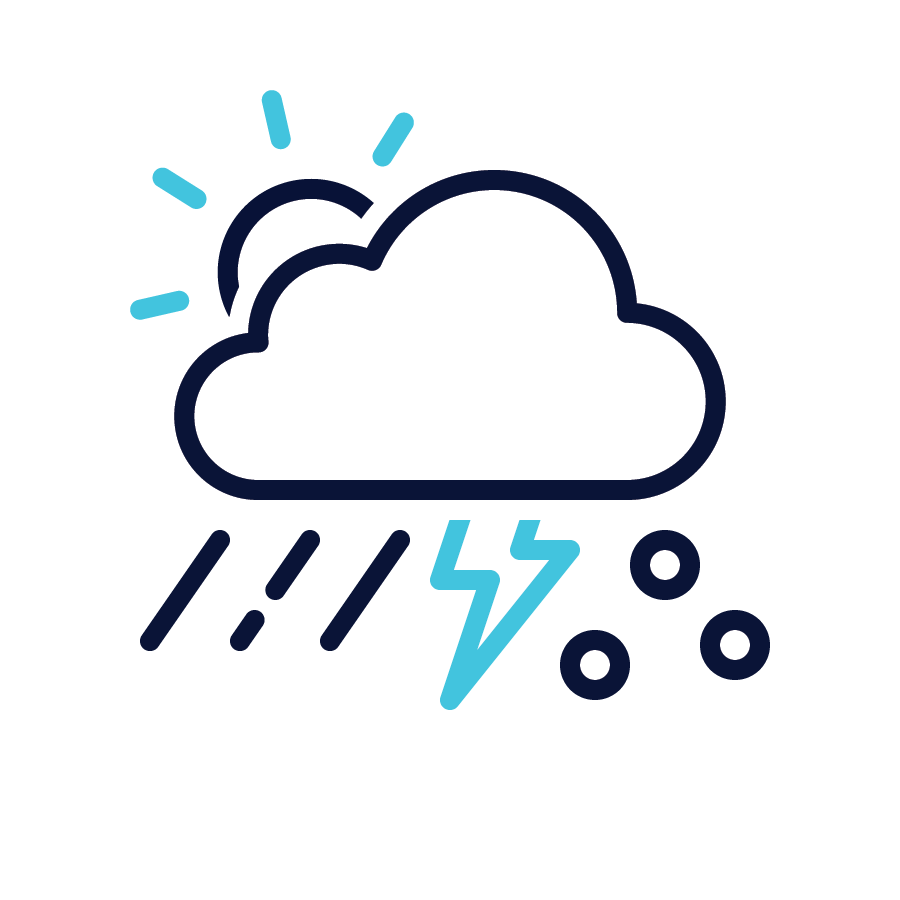
Publicly available environmental data sources to support natural catastrophe risk insights.
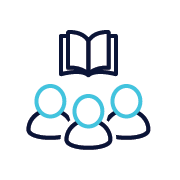
Clir’s knowledge base of specific component technology.
Deeper understanding of risks
Collaborating with customers and industry partners, Clir’s has developed a leading methodology for understanding wind and solar claims. Our risk modelling covers common causes, occurrence summary, severity assessment, fault identification, common mitigation measures and predictions of future claims. Thorough modelling enables a deeper understanding of causes of risk.
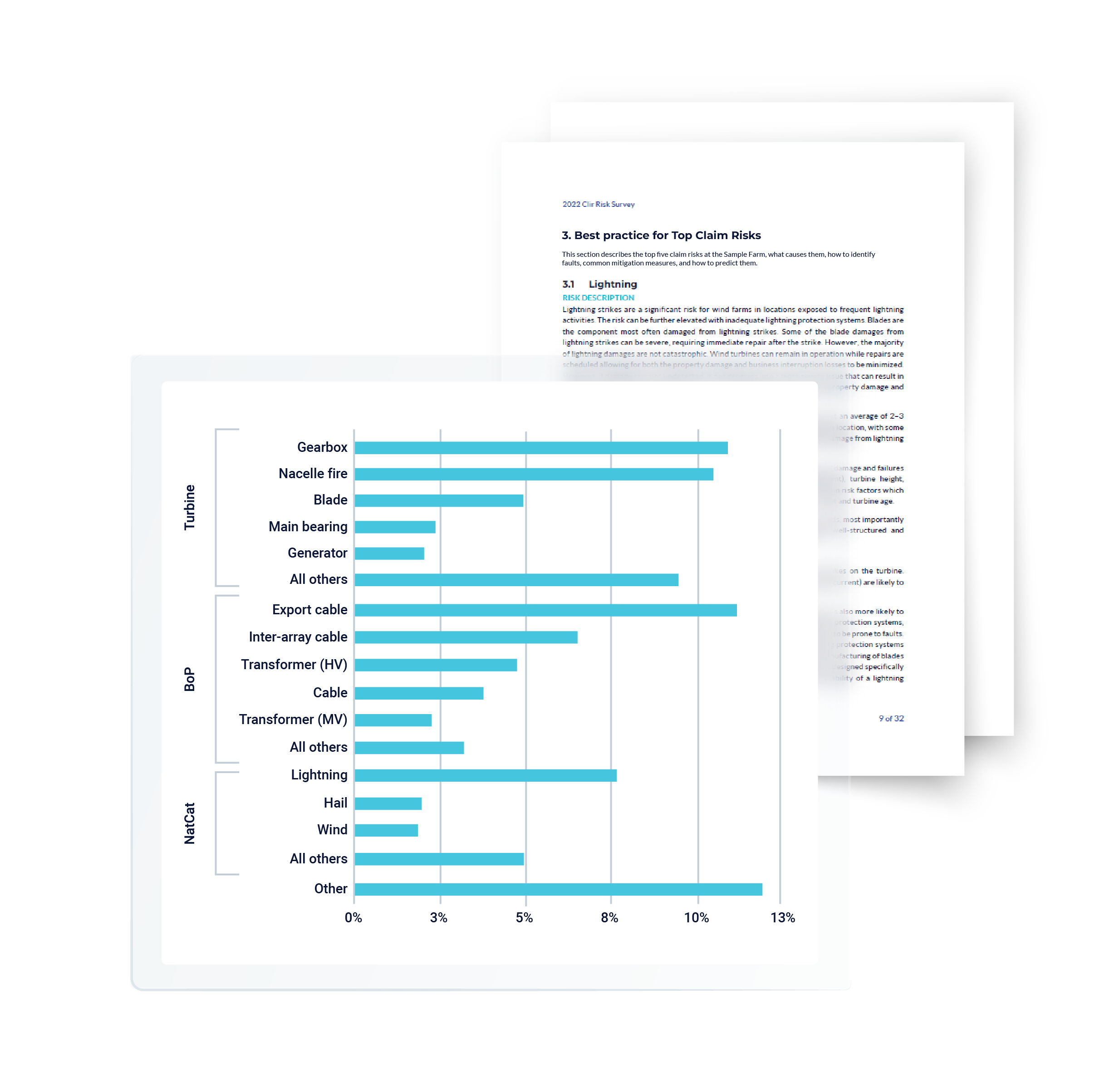
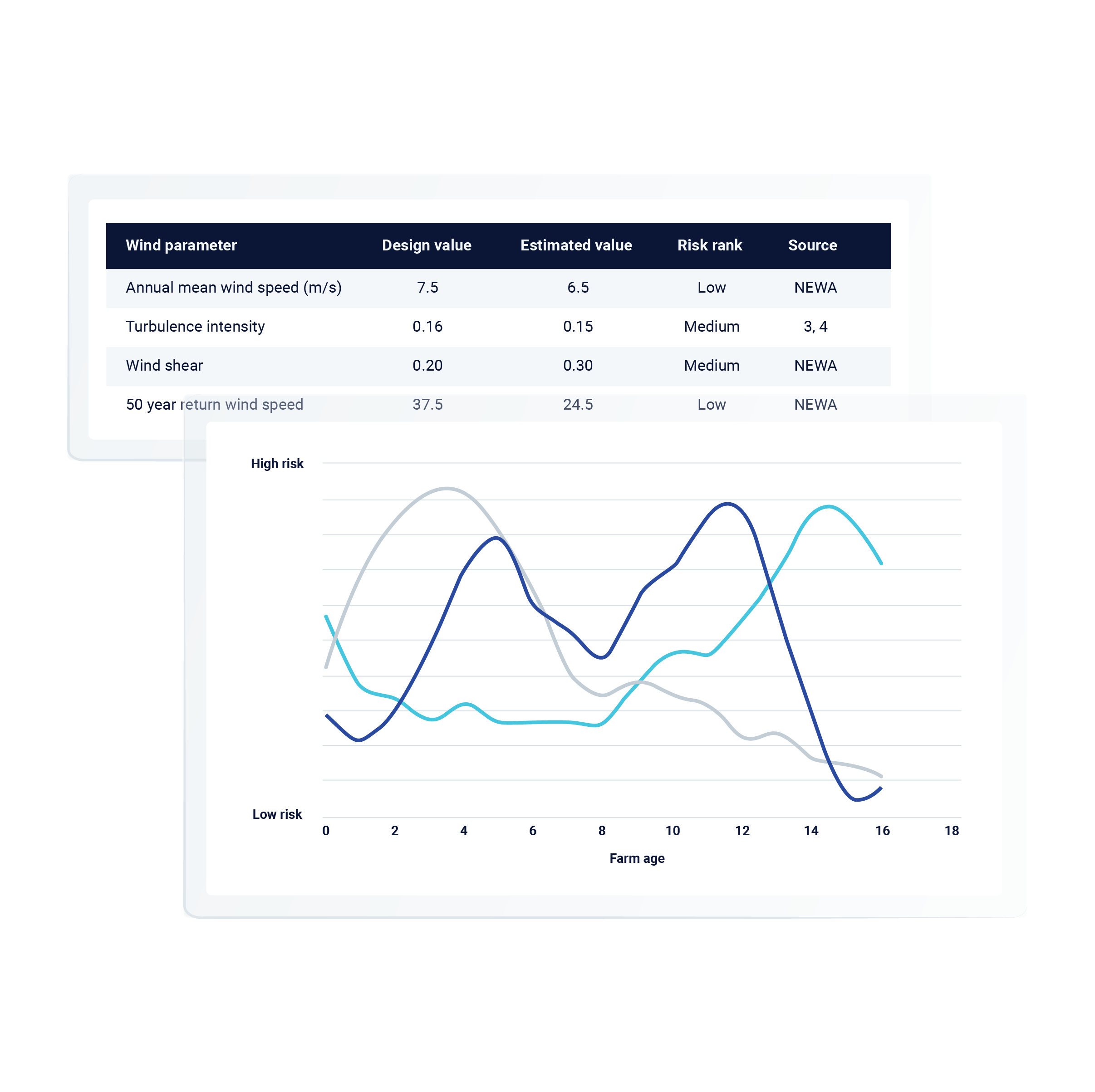
Accurate risk submissions
Factors like age, technology, OEM, service contracts and contractual structure can have a significant impact on attritional risk, and can greatly impact insurance submissions and subsequent coverage. Clir’s industry dataset can help owners and insurers better understand site-specific attritional risk rates by using technology and OEM data. This enables an accurate risk submission, adjusted to the project.
Natural catastrophe models
The insurance modelling typically used to predict natural catastrophe risks are not specific to wind and solar assets, but instead used for many industries. Clir improves risk modelling for renewable energy assets by taking into account information and issues impacting wind and solar projects, as well as project location, technology and mitigation practices. Owners and insurers leverage Clir to better understand site-specific natural catastrophe risks.
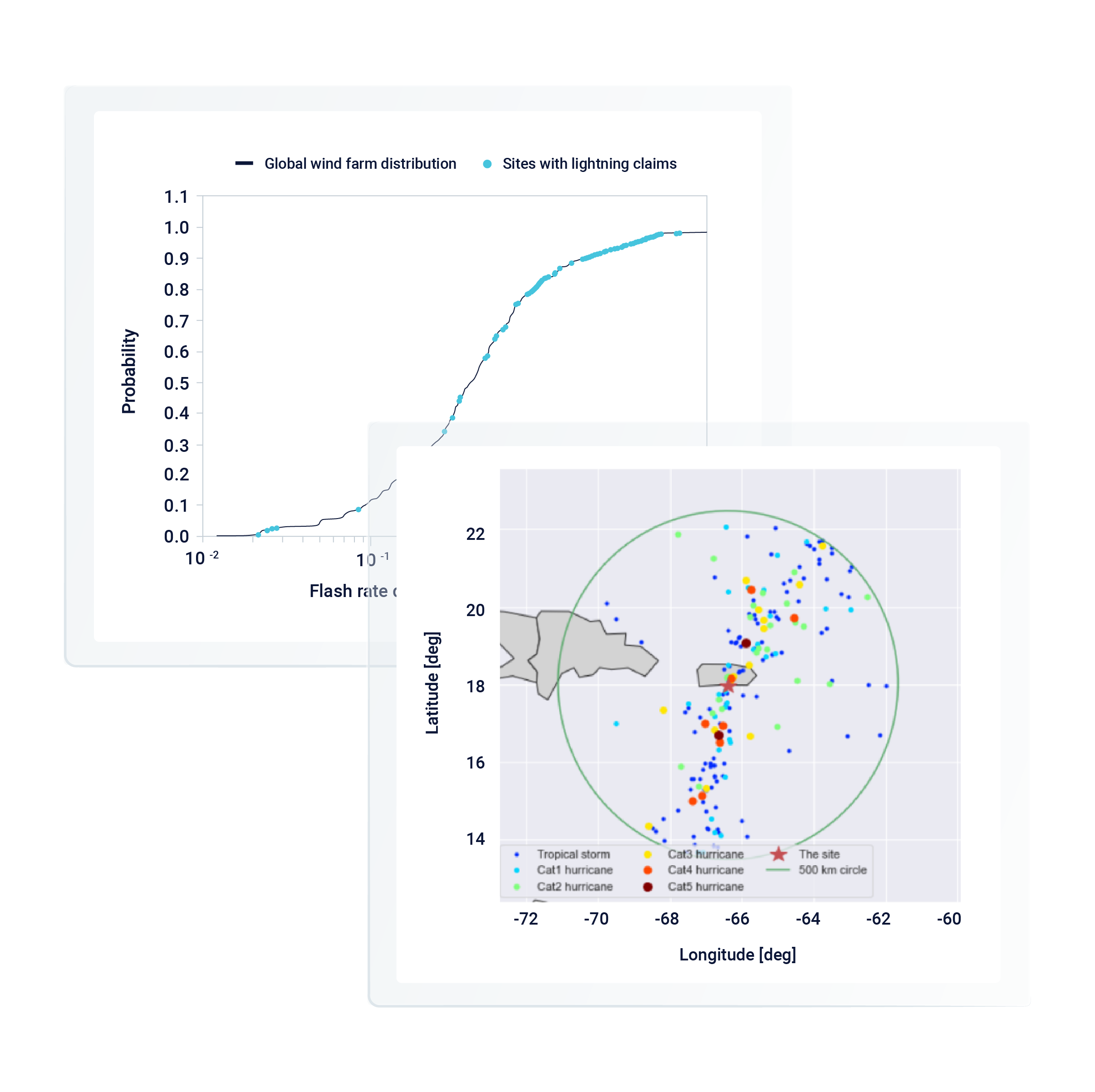
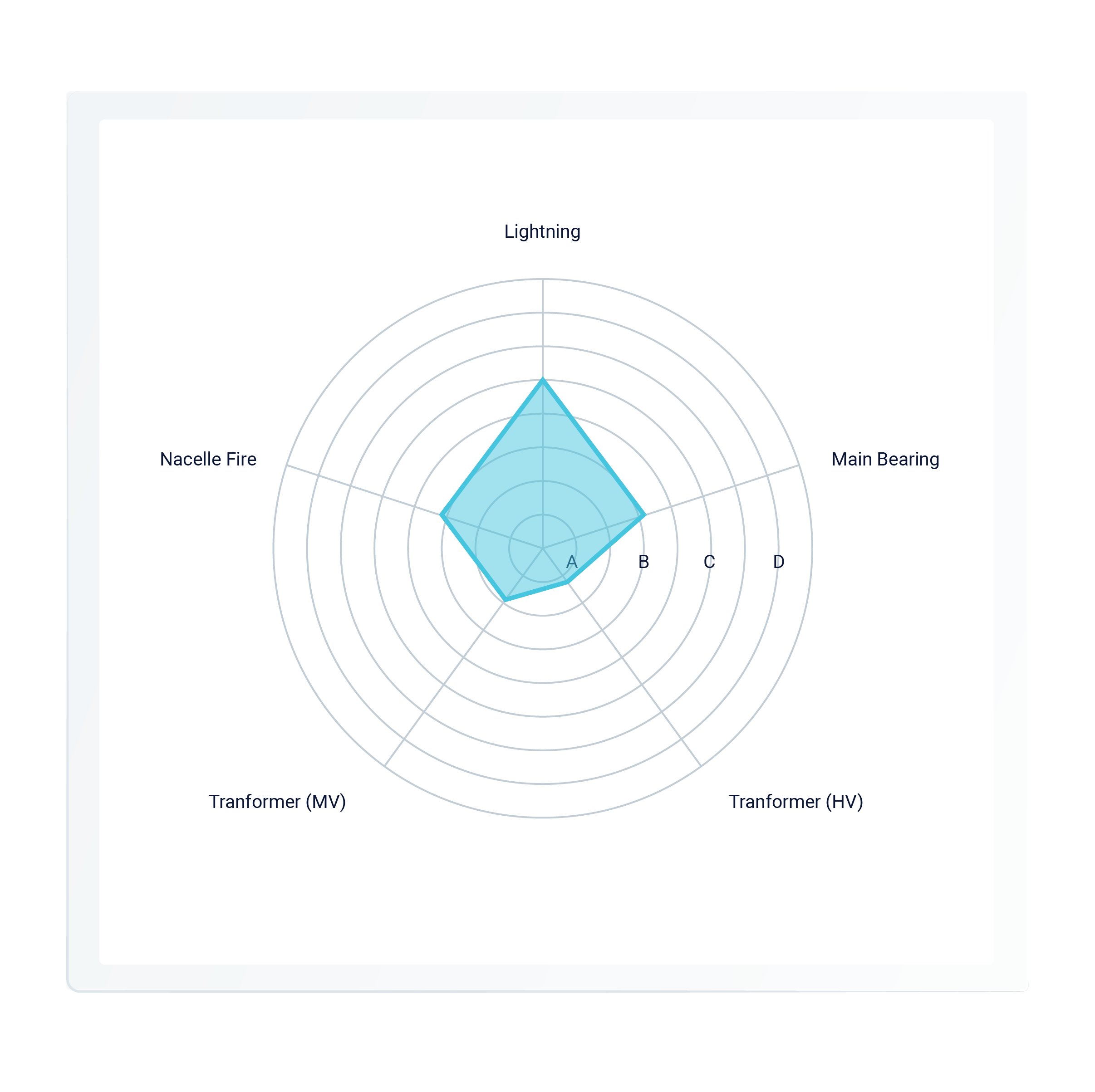
Risk severity predictions
Insurance claims are low frequency events, which makes data great for predicting if an event will occur, but poor for predicting severity. Clir’s risk modelling is further enhanced by an RFI that covers site-specific O&M contracts, technology inspection reports and service agreements. This information is used to model physical damages and business interruption, enabling a prediction of risk severities on the project.
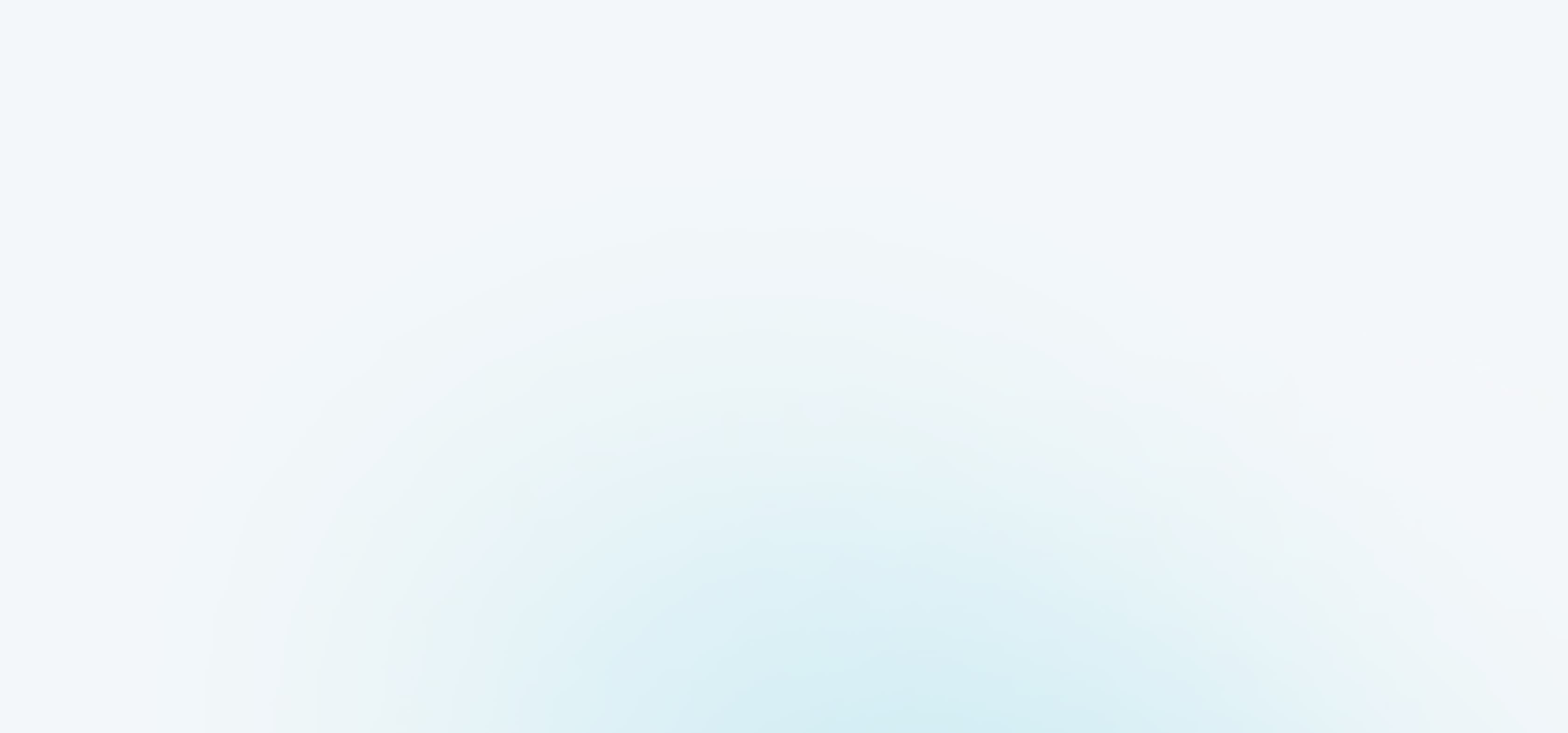